13 C-based metabolic flux analysis
Stable isotope, and in particular 13 C-based flux analysis, is the exclusive approach to experimentally quantify the integrated responses of metabolic networks. Here we describe a protocol that is based on growing microbes on 13 C-labeled glucose and subsequent gas chromatography mass spectrometric detection of 13 C-patterns in protein-bound amino acids. Relying on publicly available software packages, we then describe two complementary mathematical approaches to estimate either local ratios of converging fluxes or absolute fluxes through different pathways. As amino acids in cell protein are abundant and stable, this protocol requires a minimum of equipment and analytical expertise. Most other flux methods are variants of the principles presented here. A true alternative is the analytically more demanding dynamic flux analysis that relies on 13 C-pattern in free intracellular metabolites. The presented protocols take 5–10 d, have been used extensively in the past decade and are exemplified here for the central metabolism of Escherichia coli.
This is a preview of subscription content, access via your institution
Access options
Subscribe to this journal
Receive 12 print issues and online access
265,23 € per year
only 22,10 € per issue
Buy this article
Prices may be subject to local taxes which are calculated during checkout
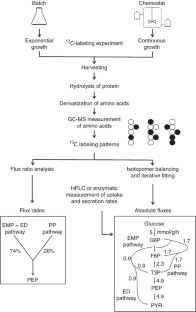
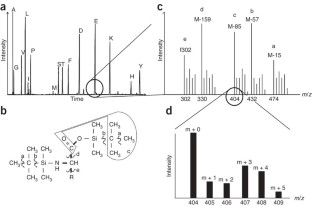
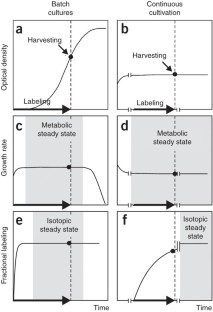
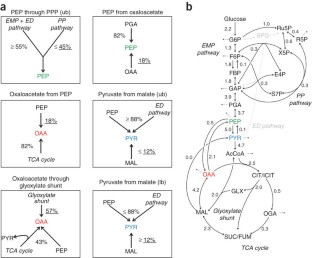
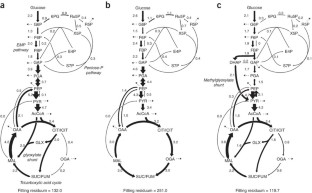
Similar content being viewed by others
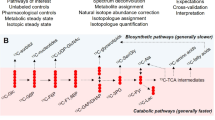
Integration of flux measurements and pharmacological controls to optimize stable isotope-resolved metabolomics workflows and interpretation
Article Open access 23 September 2019
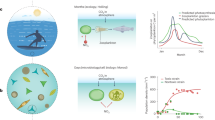
Quantitative principles of microbial metabolism shared across scales
Article 06 August 2024
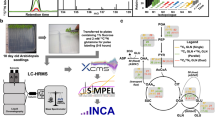
SIMPEL: using stable isotopes to elucidate dynamics of context specific metabolism
Article Open access 12 February 2024
References
- Ishii, N. et al. Multiple high-throughput analyses monitor the response of E. coli to perturbations. Science316, 593–597 (2007). ArticleCASGoogle Scholar
- Sauer, U. Metabolic networks in motion: 13 C-based flux analysis. Mol. Syst. Biol.2, 62 (2006). ArticleGoogle Scholar
- Hellerstein, M.K. In vivo measurement of fluxes through metabolic pathways: the missing link in functional genomics and pharmaceutical research. Annu. Rev. Nutr.23, 379–402 (2003). ArticleCASGoogle Scholar
- Stephanopoulos, G. Metabolic fluxes and metabolic engineering. Metab. Eng.1, 1–11 (1999). ArticleCASGoogle Scholar
- Varma, A. & Palsson, B.O. Metabolic flux balancing: Basic concepts, scientific, and practical use. Bio/Technol.12, 994–998 (1994). ArticleCASGoogle Scholar
- Wiechert, W. 13 C metabolic flux analysis. Metab. Eng.3, 195–206 (2001). ArticleCASGoogle Scholar
- Szyperski, T. 13 C-NMR, MS and metabolic flux balancing in biotechnology research. Q. Rev. Biophys.31, 41–106 (1998). ArticleCASGoogle Scholar
- Nanchen, A., Fuhrer, T. & Sauer, U. Determination of metabolic flux ratios from 13 C-experiments and gas chromatography-mass spectrometry data: protocol and principles. Methods Mol. Biol.358, 177–197 (2007). ArticleCASGoogle Scholar
- Sauer, U. High-throughput phenomics: experimental methods for mapping fluxomes. Curr. Opin. Biotechnol.15, 58–63 (2004). ArticleCASGoogle Scholar
- Wiechert, W., Möllney, M., Petersen, S. & de Graaf, A.A. A universal framework for 13 C metabolic flux analysis. Metab. Eng.3, 265–283 (2001). ArticleCASGoogle Scholar
- Fischer, E. & Sauer, U. Metabolic flux profiling of Escherichia coli mutants in central carbon metabolism using GC-MS. Eur. J. Biochem.270, 880–891 (2003). ArticleCASGoogle Scholar
- Zamboni, N., Fischer, E. & Sauer, U. FiatFlux - a software for metabolic flux analysis from 13 C-glucose experiments. BMC Bioinformatics6, 209 (2005). ArticleGoogle Scholar
- Marx, A., de Graaf, A.A., Wiechert, W., Eggeling, L. & Sahm, H. Determination of the fluxes in the central metabolism of Corynebacterium glutamicum by nuclear magnetic resonance spectroscopy combined with metabolite balancing. Biotech. Bioeng.49, 111–129 (1996). ArticleCASGoogle Scholar
- Fischer, E., Zamboni, N. & Sauer, U. High-throughput metabolic flux analysis based on gas chromatography-mass spectrometry derived 13 C constraints. Anal. Biochem.325, 308–316 (2004). ArticleCASGoogle Scholar
- Emmerling, M. et al. Metabolic flux responses to pyruvate kinase knockout in Escherichia coli . J. Bacteriol.184, 152–164 (2002). ArticleCASGoogle Scholar
- Dauner, M. & Sauer, U. GC-MS analysis of amino acids rapidly provides rich information for isotopomer balancing. Biotechnol. Prog.16, 642–649 (2000). ArticleCASGoogle Scholar
- Nöh, K. et al. Metabolic flux analysis at ultra short time scale: isotopically non-stationary 13 C labeling experiments. J. Biotechnol.129, 249–267 (2007). ArticleGoogle Scholar
- van Winden, W.A. et al. Metabolic-flux analysis of Saccharomyces cerevisiae CEN.PK113-7D based on mass isotopomer measurements of 13 C-labeled primary metabolites. FEMS Yeast Res.5, 559–568 (2005). ArticleCASGoogle Scholar
- Yuan, J., Fowler, W.U., Kimball, E., Lu, W. & Rabinowitz, J.D. Kinetic flux profiling of nitrogen assimilation in Escherichia coli . Nat. Chem. Biol.2, 529–530 (2006). ArticleCASGoogle Scholar
- Schaub, J., Mauch, K. & Reuss, M. Metabolic flux analysis in Escherichia coli by integrating isotopic dynamic and isotopic stationary 13 C labeling data. Biotechnol. Bioeng.99, 1170–1185 (2008). ArticleCASGoogle Scholar
- Hua, Q., Yang, C., Baba, T., Mori, H. & Shimizu, K. Responses of the central metabolism in Escherichia coli to phosphoglucose isomerase and glucose-6-phosphate dehydrogenase knockouts. J. Bacteriol.185, 7053–7067 (2003). ArticleCASGoogle Scholar
- Fischer, E. & Sauer, U. Large-scale in vivo flux analysis shows rigidity and suboptimal performance of Bacillus subtilis metabolism. Nat. Genet.37, 636–640 (2005). ArticleCASGoogle Scholar
- Christensen, B., Gombert, A.K. & Nielsen, J. Analysis of flux estimates based on 13 C-labeling experiments. Eur. J. Biochem.269, 2795–2800 (2002). ArticleCASGoogle Scholar
- Wittmann, C., Kiefer, P. & Zelder, O. Metabolic fluxes in Corynebacterium glutamicum during lysine production with sucrose as carbon source. Appl. Environ. Microbiol.70, 7277–7287 (2004). ArticleCASGoogle Scholar
- Hellerstein, M.K. New stable isotope-mass spectrometric techniques for measuring fluxes through intact metabolic pathways in mammalian systems: introduction of moving pictures into functional genomics and biochemical phenotyping. Metab. Eng.6, 85–100 (2004). ArticleCASGoogle Scholar
- Schwender, J. Metabolic flux analysis as a tool in metabolic engineering of plants. Curr. Opin. Biotechnol.19, 131–137 (2008). ArticleCASGoogle Scholar
- Wittmann, C. Metabolic flux analysis using mass spectrometry. Adv. Biochem. Eng. Biotechnol.74, 39–64 (2002). CASPubMedGoogle Scholar
- Gunnarsson, N., Mortensen, U.H., Sosio, M. & Nielsen, J. Identification of the Entner-Doudoroff pathway in an antibiotic-producing actinomycete species. Mol. Microbiol.52, 895–902 (2004). ArticleCASGoogle Scholar
- Fischer, E. & Sauer, U. A novel metabolic cycle catalyzes glucose oxidation and anaplerosis in hungry Escherichia coli . J. Biol. Chem.278, 46446–46451 (2003). ArticleCASGoogle Scholar
- Blank, L.M., Kuepfer, L. & Sauer, U. Large-scale 13 C-flux analysis reveals mechanistic principles of metabolic network robustness to null mutations in yeast. Genome. Biol.6, R49 (2005). ArticleGoogle Scholar
- Schütz, R., Küpfer, L. & Sauer, U. Systematic evaluation of objective functions for predicting intracellular fluxes in Escherichia coli . Mol. Syst. Biol.3, 119 (2007). Google Scholar
- Feist, A.M. & Palsson, B.O. The growing scope of applications of genome-scale metabolic reconstructions using Escherichia coli . Nat. Biotechnol.26, 659–667 (2008). ArticleCASGoogle Scholar
- Szyperski, T. Biosynthetically directed fractional 13 C-labeling of proteinogenic amino acids. An efficient analytical tool to investigate intermediary metabolism. Eur. J. Biochem.232, 433–448 (1995). ArticleCASGoogle Scholar
- Möllney, M., Wiechert, W., Kownatzki, D. & de Graaf, A.A. Bidirectional reaction steps in metabolic networks: IV. Optimal design of isotopomer labeling experiments. Biotechnol. Bioeng.66, 86–103 (1999). ArticleGoogle Scholar
- Wiechert, W., Siefke, C., de Graaf, A.A. & Marx, A. Bidirectional reaction steps in metabolic networks: II. Flux estimation and statistical analysis. Biotechnol. Bioeng.55, 118–135 (1997). ArticleCASGoogle Scholar
- Antoniewicz, M.R., Kelleher, J.K. & Stephanopoulos, G. Elementary metabolite units (EMU): a novel framework for modeling isotopic distributions. Metab. Eng.9, 68–86 (2007). ArticleCASGoogle Scholar
- van Winden, W.A., Heijnen, J.J. & Verheijen, P.J. Cumulative bondomers: a new concept in flux analysis from 2D [ 13 C, 1 H] COSY NMR data. Biotechnol. Bioeng.80, 731–745 (2002). ArticleCASGoogle Scholar
- Rantanen, A. et al. An analytic and systematic framework for estimating metabolic flux ratios from 13C tracer experiments. BMC Bioinformatics9, 266 (2008). ArticleGoogle Scholar
- Zamboni, N. et al. Transient expression and flux changes during a shift from high to low riboflavin production in continuous cultures of Bacillus subtilis . Biotechnol. Bioeng.89, 219–232 (2005). ArticleCASGoogle Scholar
- Schaub, J., Schiesling, C., Reuss, M. & Dauner, M. Integrated sampling procedure for metabolome analysis. Biotechnol. Prog.22, 1434–1442 (2006). ArticleCASGoogle Scholar
- Antoniewicz, M.R. et al. Metabolic flux analysis in a nonstationary system: fed-batch fermentation of a high yielding strain of E. coli producing 1,3-propanediol. Metab. Eng.9, 277–292 (2007). ArticleCASGoogle Scholar
- Monod, J. Récherches sur la croissance des cultures bactériennnes (Hermann et Compagnie Ed, Paris, 1942). Google Scholar
- Kleijn, R.J. et al. 13 C-labeled gluconate tracing as a direct and accurate method for determining the pentose phosphate pathway split ratio in Penicillium chrysogenum . Appl. Environ. Microbiol.72, 4743–4754 (2006). ArticleCASGoogle Scholar
- Petersen, S. et al. In vivo quantification of parallel and bidirectional fluxes in the anaplerosis of Corynebacterium glutamicum . J. Biol. Chem.275, 35932–35941 (2000). ArticleCASGoogle Scholar
- Stryer, L. Biochemistry 4th edn. 483–491 (Freeman and Company, New York, 1995).
- Dauner, M. et al. Intracellular carbon fluxes in riboflavin-producing Bacillus subtilis during growth on two-carbon substrate mixtures. Appl. Environ. Microbiol.68, 1760–1771 (2002). ArticleCASGoogle Scholar
- Blank, L.M., Lehmbeck, F. & Sauer, U. Metabolic-flux and network analysis in fourteen hemiascomycetous yeasts. FEMS Yeast Res.5, 545–558 (2005). ArticleCASGoogle Scholar
- Fischer, E., Zamboni, N. & Sauer, U. High-throughput metabolic flux analysis based on gas chromatography-mass spectrometry derived 13C constraints. Anal. Biochem.325, 308–316 (2004). ArticleCASGoogle Scholar
- Kleijn, R.J. et al. Metabolic flux analysis of a glycerol-overproducing Saccharomyces cerevisiae strain based on GC-MS, LC-MS and NMR-derived C-13-labelling data. FEMS Yeast Res.7, 216–231 (2007). ArticleCASGoogle Scholar
- Kummel, A., Panke, S. & Heinemann, M. Systematic assignment of thermodynamic constraints in metabolic network models. BMC Bioinformatics7, 512 (2006). ArticleGoogle Scholar
- Herrgard, M.J. et al. A consensus yeast metabolic network reconstruction obtained from a community approach to systems biology. Nat. Biotechnol.26, 1155–1160 (2008). ArticleCASGoogle Scholar
- Oh, Y.K., Palsson, B.O., Park, S.M., Schilling, C.H. & Mahadevan, R. Genome-scale reconstruction of metabolic network in Bacillus subtilis based on high-throughput phenotyping and gene essentiality data. J. Biol. Chem.282, 28791–28799 (2007). ArticleCASGoogle Scholar
- Feist, A.M. et al. A genome-scale metabolic reconstruction for Escherichia coli K-12 MG1655 that accounts for 1260 ORFs and thermodynamic information. Mol. Syst. Biol.3, 121 (2007). ArticleGoogle Scholar
- Schilling, C.H. et al. Genome-scale metabolic model of Helicobacter pylori 26695. J. Bacteriol.184, 4582–4593 (2002). ArticleCASGoogle Scholar
- Oliveira, A.P., Nielsen, J. & Forster, J. Modeling Lactococcus lactis using a genome-scale flux model. BMC Microbiol.5, 39 (2005). ArticleGoogle Scholar
- Cannizzaro, C., Christensen, B., Nielsen, J. & von Stockar, U. Metabolic network analysis on Phaffia rhodozyma yeast using 13 C-labeled glucose and gas chromatography-mass spectrometry. Metab. Eng.6, 340–351 (2004). ArticleCASGoogle Scholar
- Fuhrer, T., Fischer, E. & Sauer, U. Experimental identification and quantification of glucose metabolism in seven bacterial species. J. Bacteriol.187, 1581–1590 (2005). ArticleCASGoogle Scholar
- Schmidt, K., Carlsen, M., Nielsen, J. & Villadsen, J. Modeling isotopomer distributions in biochemical networks using isotopomer mapping matrices. Biotechnol. Bioeng.55, 831–840 (1997). ArticleCASGoogle Scholar
- Arita, M. In silico atomic tracing by substrate-product relationships in Escherichia coli intermediary metabolism. Genome Res.13, 2455–2466 (2003). ArticleCASGoogle Scholar
- Pitkänen, E., Åkerlund, A., Rantanen, A., Jouhten, P. & Ukkonen, E. ReMatch: a web-based tool to construct, store and share stoichiometric metabolic models with carbon maps for metabolic flux analysis. J. Integr. Bioinform.5, 102 (2008). ArticleGoogle Scholar
- Dauner, M., Bailey, J.E. & Sauer, U. Metabolic flux analysis with a comprehensive isotopomer model in Bacillus subtilis . Biotechnol. Bioeng.76, 144–156 (2001). ArticleCASGoogle Scholar
- Pramanik, J. & Keasling, J.D. Effect of Escherichia coli biomass composition on central metabolic fluxes predicted by a stoichiometric model. Biotechnol. Bioeng.60, 230–238 (1998). ArticleCASGoogle Scholar
- Guy, R.D., Fogel, M.L. & Berry, J.A. Photosynthetic fractionation of the stable isotopes of oxygen and carbon. Plant Physiol.101, 37–47 (1993). ArticleCASGoogle Scholar
- Srere, P.A. Citric acid cycle redux. Trends Biochem. Sci.15, 411–412 (1990). ArticleCASGoogle Scholar
- Antoniewicz, M.R., Kelleher, J.K. & Stephanopoulos, G. Accurate assessment of amino acid mass isotopomer distributions for metabolic flux analysis. Anal. Chem.79, 7554–7559 (2007). ArticleCASGoogle Scholar
- Zamboni, N. in Topics in Current Genetics (eds. J. Nielsen & M. Jewett) (Springer, Berlin, 2007). Google Scholar
- Isermann, N. & Wiechert, W. Metabolic isotopomer labeling systems. Part II: structural flux identifiability analysis. Math. Biosci.183, 175–214 (2003). ArticleCASGoogle Scholar
- Rantanen, A., Mielikainen, T., Rousu, J., Maaheimo, H. & Ukkonen, E. Planning optimal measurements of isotopomer distributions for estimation of metabolic fluxes. Bioinformatics22, 1198–1206 (2006). ArticleCASGoogle Scholar
- Sambrook, T. & Russell, D.W. in Molecular Cloning: A Laboratory ManualVol 3, A2.2 (Cold Spring Harbor Press, Cold Spring Harbor, NY, 2001). Google Scholar
- Bailey, J.E. & Ollis, D.F. in Biochemical Engineering Fundamentals 2nd edn. 373–456 (McGraw-Hill, Singapore, 1986). Google Scholar
- Wahl, S.A., Dauner, M. & Wiechert, W. New tools for mass isotopomer data evaluation in (13)C flux analysis: mass isotope correction, data consistency checking, and precursor relationships. Biotechnol. Bioeng.85, 259–268 (2004). ArticleCASGoogle Scholar
- Pázman, A. Nonlinear Statistical Models (Kluwer Academic Publishing, New York, 1993). BookGoogle Scholar
- Gottschalk, G. Bacterial Metabolism 2nd edn. 185 (Springer-Verlag, New York, 1986). BookGoogle Scholar
- Fong, S.S., Nanchen, A., Palsson, B.O. & Sauer, U. Latent pathway activation and increased pathway capacity enable Escherichia coli adaptation to loss of key metabolic enzymes. J. Biol. Chem.281, 8024–8033 (2006). ArticleCASGoogle Scholar
- Wiechert, W. & de Graaf, A.A. Bidirectional reaction steps in metabolic networks: I. Modeling and simulation of carbon isotope labeling experiments. Biotechnol. Bioeng.55, 101–117 (1997). ArticleCASGoogle Scholar
- Wiechert, W., Mollney, M., Isermann, N., Wurzel, M. & de Graaf, A.A. Bidirectional reaction steps in metabolic networks: III. Explicit solution and analysis of isotopomer labeling systems. Biotechnol. Bioeng.66, 69–85 (1999). ArticleCASGoogle Scholar
Acknowledgements
We thank Katharina Nöh and Wolfgang Wiechert for support with 13CFLUX and comments on the respective protocol parts presented in this protocol, as well as Roelco J. Kleijn, Dominik Heer, Julian Schnidder, and Daniel Heine for constructive comments on the script.
Author information
Authors and Affiliations
- Institute of Molecular Systems Biology, ETH Zurich, Zurich, 8093, Switzerland Nicola Zamboni, Sarah-Maria Fendt, Martin Rühl & Uwe Sauer
- PhD Program Systems Biology of Complex Diseases, ETH Zurich, Zurich, Switzerland Sarah-Maria Fendt
- PhD Program Molecular Life Sciences, ETH Zurich, Zurich, Switzerland Martin Rühl
- Nicola Zamboni